1.3.3. 模型性能
您可以借助下表估计模型的性能。它包含依赖于 FLOP 和参数的 OAK 设备上模型的 FPS 估计。
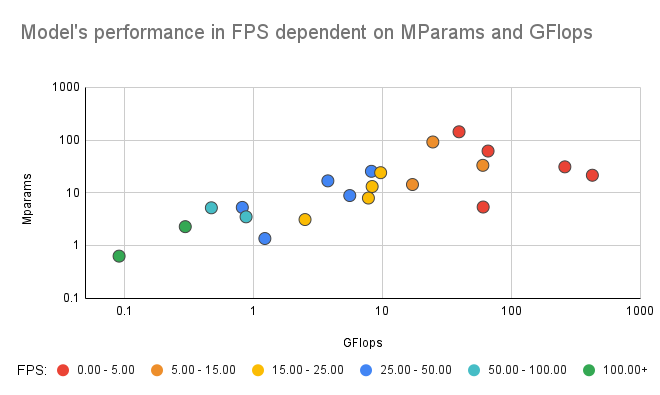
您可以在此表 中找到更详细的通用模型 FPS评估。
1.3.4. AI视觉任务
我们为许多不同的AI视觉任务提供开源示例和演示,例如:
Object detection 模型提供所有检测到的对象的边界框、置信度和标签。 示例: MobileNet, Yolo, EfficientDet, Palm detection.
Landmark detection 模型提供对象的特征/关键点。 示例: Human pose, hand landmarks, and facial landmarks.
Semantic segmentation 模型为每个像素提供标签/类别。 示例: Person segmentation, multiclass segmentation, road segmentation.
Classification 模型提供分类标签和对该标签的置信度。 示例: EfficientNet, Tensorflow classification, fire classification, emotions classification.
Recognition 模型提供可用于识别或识别特征本身的字节数组。 示例: Face recognition, person identification, OCR, license plate recognition.
还有许多其他 AI 视觉任务不属于上述任何类别, 例如 crowd counting, monocular depth estimation, gaze estimation, 或 age/gender estimation.
上面的所有演示都在彩色/灰度帧上运行。 其中许多视觉任务可以与深度感知(在OAK相机本身上) 融合 , 从而释放 Spatial AI 的力量。